About UWORCS
UWORCS stands for University of Western Ontario Research in Computer Science. UWORCS is our annual departmental student conference and provides a great opportunity to develop your presentation skills in front of a friendly audience of your peers and faculty members.
Your participation is needed to make this event a success. Please email Andrew Bloch-Hansen at ablochha@uwo.ca for more details.
Subjects
Presenters
Registered Attendees
Keynote Speaker
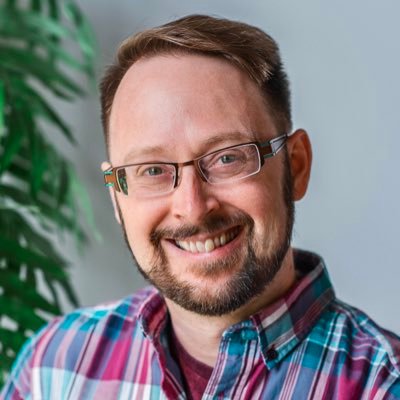
Patrick Pilarski
Dr. Patrick Pilarski is a Canada CIFAR Artificial Intelligence Chair (Amii), past Canada Research Chair in Machine Intelligence for Rehabilitation, and an Associate Professor in the Division of Physical Medicine and Rehabilitation, Department of Medicine, University of Alberta. He is a Fellow of the Alberta Machine Intelligence Institute (Amii), co-leads the Bionic Limbs for Improved Natural Control (BLINC) Laboratory, and is a principal investigator with the Reinforcement Learning and Artificial Intelligence Laboratory (RLAI), and also iSMART, at the University of Alberta. Dr. Pilarski's research interests include reinforcement learning and decision making, artificial intelligence, real-time machine learning, human-machine interaction, intelligence amplification, rehabilitation technology, and assistive robotics. He leads the Amii Adaptive Prosthetics Program—an interdisciplinary initiative focused on creating intelligent artificial limbs to restore and extend abilities for people with amputations. As part of this research, Dr. Pilarski has developed and made prominent machine learning techniques for continual sensorimotor control and prediction learning on prosthetic devices.
Talk Title
Reimagining Human Interaction with Rehabilitation Technologies (naturally, by way of continual machine learning)
As powered upper-limb prostheses (artificial limbs) have advanced through technological innovation, new surgical capabilities, and enhanced clinical care and training paradigms, their degree of integration with their human users has changed dramatically. Tighter coupling of machines with users, combined with a rapid step-change in available computing capabilities, have made it now possible for prosthetic systems to adapt during both training and deployment. More strongly: modern prosthetic technology has the necessary preconditions to construct many key elements of its operation from experience obtained during deployed interactions with users over extended periods of time. This talk will focus on the use of artificial intelligence and machine learning methods to improve the control of and feedback from myoelectric prostheses, and present a bold vision for an alternative approach to thinking about the emerging relationship between a person and their assistive technology. This vision is rooted in continual learning on the part of both the human and the machine, and will be connected to concrete examples of work from the BLINC Lab at the University of Alberta on developing upper-limb prosthetic interfaces using techniques and viewpoints from the field of reinforcement learning.
Dr. Pilarski's keynote speech will take place on Tuesday, April 9th at 12:15pm, in Middlesex College room MC110. Please join us to welcome Dr. Pilarski to the 31st Annual Conference - UWORCS 2024.
Meeting ID: 978 5456 1095
Meet and Greet
We are hosting a Meet-And-Greet on April 9 from 8:00am to 9:00am before the presentations begin. This is a great networking opportunity and it may help you decide which presentations to attend! All registered attendees can pick up their name tag, and a limited number of purple Western lanyards will be available on a first-come first-served basis. A small selection of snacks will be available.
Trivia
At 2:00pm, shortly after setting out the lunch food, we will begin our trivia session in MC316. We will play one round of trivia for each of our presentation sessions using questions provided by our presenters that are based on their talks. The top two scorers in each session will receive prizes!
Since each trivia round will only feature trivia questions from a single presentation session, your chances of winning these prizes will increase if you attend multiple presentations from the same session. Kahoot is an online game that can been accessed using a web-browser or through the mobile app.
In order to ensure that the correct participants receive the prizes, please use your real name during the Kahoot session. Presenters who participate in the trivia round corresponding to their own presentation session will receive a penalty to their score of 1500 points to account for answering their own trivia questions correctly.
Frequently Asked Questions
Here are the FAQs for UWORCS 2024.
-
Who can attend?
Computer science faculty, graduate students, and undergraduate students are invited to attend to listen to the presentations. Students from other faculties are also welcome.
-
Is there a registration fee?
No registration fee.
-
What sort of research can be presented?
The more you care about a subject the better your talk will probably be. Choose something that you've personally worked on during your grad/undergrad thesis studies, or even a course project with a research flavour. You can even present ongoing research. UWORCS is a great opportunity to practice explaining whatever work you are most proud of.
-
How should I prepare my talk?
Each presentation should be 20 minutes long with an additional 7 minutes for questions.
Presentations should clearly state the research problem and motivation, should include relevant facts/data/analysis to support the research strategy, should be accessible to an audience with an undergraduate-level background in computer science, and should have a smooth flow of ideas. -
Does this fulfill my yearly PhD seminar?
Yes. PhD students in their 3rd and 4th years can present their current research and have it count towards their yearly seminar requirement (692).
-
Will there be session prizes?
Yes, each session will have a cash prize for the best presentation. The number and size of the prizes will be determined once we have made the schedule.
-
How does session judging/chairing work?
Each presentation will be given a score out of 50 by each of three faculty members (for a total score out of 150), based mainly on presentation quality and clarity. The highest score out of 150 for each session is awarded the best presentation award. Feedback from the judges will be forwarded to each presenter after the event is over. Session chairs announce each speaker and ensure that the talks stay on schedule.
-
Is lunch included?
Yes, lunch will be available to all participants and registered attendees. We have not yet set a menu, but we will ensure that an appropriate variety of lunch options are provided. In addition, coffee and snacks will be provided throughout the day.
Subjects
UWORCS 2024 involves talks that are judged by faculty members and senior students and prizes are awarded to top presenters
in a variety of categories including the following subjects. Topics of interest include, but are not limited to:
Team
The team for 2024 year
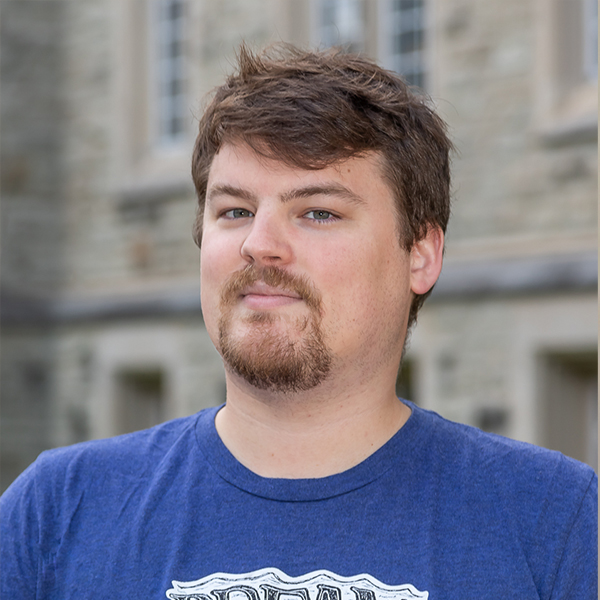
Andrew Bloch-Hansen
Conference Chair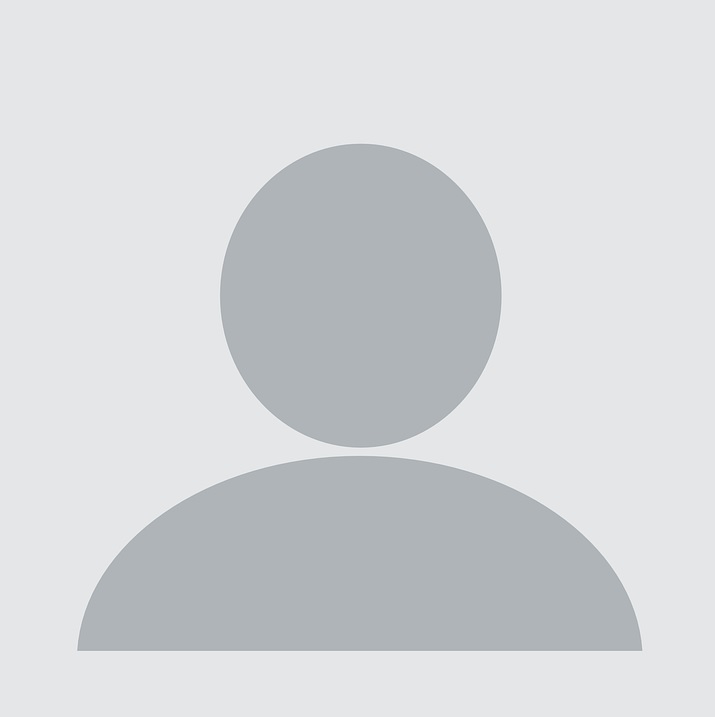
Regina Liang
Web Master & Organizing Committee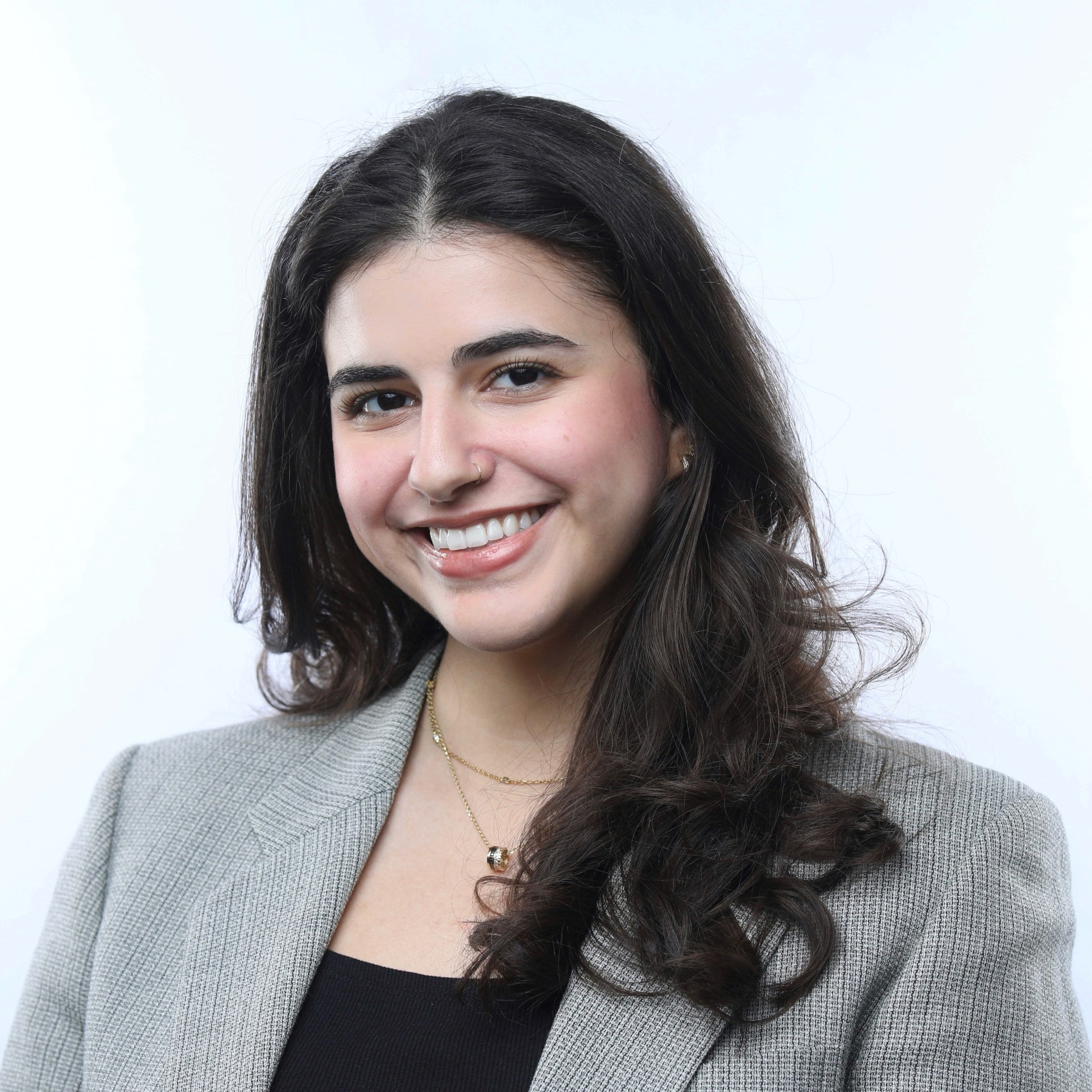
Joud El-Shawa
Organizing CommitteePresenters
These are the presenters and their topics.
Waiting for your participation!
-
Enhancing Understanding of Image Memorability: A Machine-Learning Based Approach Using Categorical Data
Johann Cardenas
Artificial IntelligenceImage memorability is the likelihood of recalling an image you have seen before. Humans predictions on what images are memorable are weakly correlated with experimental studies; aesthetics, popularity, and interestingness have also all shown to be weak predictors of image memorability. Despite this, image memorability is consistent across individuals and age groups, which suggests that memorability is an intrinsic property of images. Studies have also shown that semantic category plays a role in memorability. In this study we fine-tune machine-learning models for image memorability on MemCat, the first category-based memorability dataset, to analyze the differences in memorability within and between semantic categories. We also measure the correlation between our predictions and experimental adolescent memorability scores using the Memoir dataset to the analyze the consistency of our predictions across age groups. Our results showed improved performance after fine-tuning memorability models on category-specific data and similar model predictions had similar correlations for both adult and adolescent data.
-
A Unified Solution for Privacy and Communication Efficiency in Vertical Federated Learning
Ganyu Wang
Distributed Systems and ApplicationsVertical Federated Learning (VFL) is a collaborative machine learning paradigm that enables multiple participants to jointly train a model on their private data without sharing it. To make VFL practical, privacy security and communication efficiency should both be satisfied. Recent research has shown that Zero-Order Optimization (ZOO) in VFL can effectively conceal the internal information of the model without adding costly privacy protective add-ons, making it a promising approach for privacy and efficiency. However, there are still two key problems that have yet to be resolved. First, the convergence rate of ZOO-based VFL is significantly slower compared to gradient-based VFL, resulting in low efficiency in model training and more communication round, which hinders its application on large neural networks. Second, although ZOO-based VFL has demonstrated resistance to state-of-the-art (SOTA) attacks, its privacy guarantee lacks a theoretical explanation. To address these challenges, we propose a novel cascaded hybrid optimization approach that employs a zeroth-order (ZO) gradient on the most critical output layer of the clients, with other parts utilizing the first-order (FO) gradient. This approach preserves the privacy protection of ZOO while significantly enhancing convergence. Moreover, we theoretically prove that applying ZOO to the VFL is equivalent to adding Gaussian Mechanism to the gradient information, which offers an implicit differential privacy guarantee. Experimental results demonstrate that our proposed framework achieves similar utility as the Gaussian mechanism under the same privacy budget, while also having significantly lower communication costs compared with SOTA communication-efficient VFL frameworks.
-
MavSec: A safer version of MavLink
Chongju Mai
Software EngineeringThe MavLink protocol is a lightweight communication protocol used for communication between unmanned aerial vehicles (UAVs) and the ground control station (GCS). The contents of the MavLink payload might include sensitive information, including mission details and the geographical coordinates of the drone. Nonetheless, due to the lack of encryption support in the MavLink protocol, the payload can be readily obtained and modified by an attacker. This study presents a novel protocol called MavLink Secure (MavSec) that offers native support for encryption. Furthermore, we have also incorporated the implementation of secure key exchange. Then, our proposed protocol was tested with various encryption algorithms (AES, RC4, ChaCha20, TWINE, PRESENT) implemented in Python. Next, we proceed to evaluate the performance metrics like peak memory use, average encode time, average decode time, average latency, and average process time. The results of experiments indicate that ChaCha20 has better overall performance in comparison to other encryption algorithms. The integration of ChaCha20 with MavSec has resulted in enhanced levels of confidentiality, integrity, and authenticity compared to the unprotected MavLink protocol.
-
Wave Function Approximation: Performant Level Generation for Games
Mathias Babin
GamesThis work presents a novel approach to integrating artificial neural networks (ANNs) into the wave function collapse (WFC) algorithm for more efficient level generation. WFC is a texture synthesis algorithm that has shown promising results for generating textures as well as entire 2D and 3D levels for video games. In previous works, we have shown how this algorithm's heuristic could be replaced by an ANN trained to factor playability into its solutions. This work builds on this approach by introducing new methods aimed at the algorithm's performance, specifically, the use of AI-based function approximation to relieve the heavy computational needs of its constraint satisfaction steps. We also discuss how we can use the marching squares/cubes algorithm to reduce both the input and output space of our model, leading to further performance benefits. Lastly, we discuss how our methods here, as well as those presented in our previous works, can be extended beyond regular 2D grids for results which appear more natural and realistic.
-
A Transformer for Converting Infrastructural Config Files for Use in Multi-Cloud Settings
Faranak Adinehzadeh
Software EnginerringThis project aims to streamline the administration of cloud infrastructure for businesses by developing a tool that acts as a universal adapter for the configuration languages used by various cloud platforms, such as AWS or Azure. This solution will enable the translation and conversion of cloud configuration instructions into a standard format, facilitating effortless migration and management across different cloud services, ultimately leading to more efficient and adaptable cloud operations for expanding companies.
-
Towards a Framework for Enhancing Cloud Security in CI/CD Pipelines Using Blockchain Technology
Sabbir Muhammad Saleh
Software EngineeringCloud security is an important concern in cloud environments. Several sectors have witnessed recent cyber-attacks on their computing systems. Our research focuses on security issues within Continuous Integration and Deployment (CI/CD) pipelines in a cloud environment as a reaction to breaches in tools such as GitHub Actions (GHA), Harbor Registry, Docker Container, etc. Previous studies have shown that CI/CD pipeline tools are vulnerable, as evidenced by image manipulation, unauthorized access, lack of authentication, etc. This research proposes a blockchain-based solution combining Hyperledger Fabric and Jenkins to enhance CI/CD pipeline security. The goal is to emphasize secure software practices such as integrating threat modelling and adherence to coding standards. It will employ tools to automate security testing and detect known vulnerabilities.
-
Enhancing the Cloud Security through Topic Modeling
Sabbir Muhammad Saleh
Software EngineeringIn an age where security is a constant threat to the digital world, protecting the cloud environment is crucial. The inevitable, ever-evolving cyber-attacks (ransomware, supply chain attack) make cloud security innovations necessary. This thesis is motivated by applying Natural Language Processing (NLP) methodologies, such as Topic Modelling, to analyze security data and mitigate future attacks. This research aims to utilize the Latent Dirichlet Allocation (LDA) and Latent Semantic Analysis (LSA) to analyze fragmented and scattered security-related text data, for example, partial incident reports, truncated logs, or isolated pieces of information (e.g., version changelogs). Utilizing synonyms (for example, protection, risk, threat, virus, spyware, etc.) and associated terminology (such as encryption, phishing, hacking, identity theft, etc.), we aim to encounter hidden and uncovered insights, classify the incidents, and strengthen security intelligence. The research implementation will result in improved incident response, mitigating risks, heightened security awareness, and substantially advancing cloud security practices. This implementation of Topic Modelling intends to strengthen vulnerability detection by seamlessly integrating with the CI/CD pipeline just before deployment, thereby enhancing cloud security.
-
Optimizing Cooper's Algorithm for Quantifier Elimination
Christopher Frank Stephan Maligec
Computer AlgebraKnowing if there are integer points in polyhedra can be used to answer questions like how many memory locations are accessed by a nest of for-loops (for-loops inside for-loops), or performing dependence analysis for for-loop nests, which can determine whether these nests can be parallelized or not (Jing and Moreno Maza, 2017). Polyhedra can be described in either of two ways: as sets of vertices and rays or as systems of linear inequalities; we will focus on the latter representation. If the inequalities can be described as formulas of Presburger Arithmetic, then using quantifier elimination to eliminate variables from such systems of linear inequalities will determine if they have solutions in the integers or not. One of the main algorithms for such quantifier elimination is Cooper's Algorithm (Cooper, 1972). We hope to optimize this by using techniques from polyhedral geometry, Hermite Normal Form, and the Omega Test (Pugh, 1991). A future implementation of this optimization is also planned.
-
Integrative Data Analysis to Uncover Transcription Factors Involved in Gene Dysregulation of Nine Autoimmune and Inflammatory Diseases
Nader Hosseini Naghavi
BioinformaticsAutoimmune and inflammatory diseases are a group of > 80 complex diseases caused by loss of tolerance of the immune system for self-antigens. The biological mechanisms of autoimmune diseases are largely unknown, preventing the development of effective treatment options. Integrative analysis of genome-wide association studies and epigenetic data has shown that the risk variants of autoimmune diseases are enriched in epigenetic regions of immune cells, supporting their role in gene regulation. However, we still lack a systematic and unbiased identification of transcription factors (TF) involved in disease gene regulation.
We hypothesized that for some of the disease-relevant TFs, their binding to DNA is affected at multiple genomic sites rather than a single site, and these effects are cell-type specific. In this study, we developed a statistical approach to assess enrichment of TFs in being affected by disease risk variants at multiple sites. We used genetic association data of nine autoimmune diseases (obtained from Immunochip) and identified 99% credible interval (CI) SNPs for each trait. We then integrated CI SNPs and DNase-I footprinting data of 376 samples comprising 35 unique cell types, and employed a probabilistic model to identify the CI SNPs that are likely to change binding probability of certain TFs at specific cell types. Finally, for each TF (out of 1,372 TFs), we used Fisher's Exact test to assess whether CI SNPs show enrichments in terms of changing the binding probability of that TF at multiple sites (FDR < 10%).
Our analysis resulted in identification of significantly enriched TFs and their relevant cell types for each trait. The number of prioritized TFs in immune cell types varied between 1 and 14 for seven autoimmune diseases. For the two other traits, we did not find any significant TFs, likely due to a lack of statistical power because of their smaller sample sizes in Immunochip data. Our analysis identified some TFs previously known to be relevant to autoimmune diseases (e.g. Ahr:Arnt for rheumatoid arthritis and SPI-B for multiple sclerosis), and some other less studied new TFs. The enriched cell types also varied across the traits (e.g., CD8 and Mobilized CD4 T cells for rheumatoid arthritis, and CD56 and Mobilized CD4 T cells for multiple sclerosis). Our ChromHMM analysis proved that our predicted DNase-I footprinting sites are active enhancers or promoters in the relevant cell types. Additionally, our GREAT pathway analysis showed that the majority of the significant biological pathways are immune-related, an example of which is B cell adhesion pathway in multiple sclerosis.
Although we applied our framework to the data from autoimmune diseases, our model is general and can be applied to genetics association data from various common complex diseases. This will identify disease-relevant TFs and their relevant cell types, and will facilitate discovering specific gene regulatory mechanisms of complex diseases. -
A Modified Hopfield Network for the K-Median Problem
Andrew Bloch-Hansen
Theoretical Computer ScienceThe K-Median problem is a classical clustering problem where given n locations one wants to select k locations such that the total distance between every non-selected location and its nearest selected location is minimized. We present a modified Hopfield Network for the problem and compare it against several local search algorithms.
-
Deep Learning Based Malapps Detection in Android Powered Mobile Cyber-Physical System
Moinul Sayed
Artificial IntelligenceThe Mobile Cyber-Physical System (MCPS) integrates the mobility of various smart devices to exchange information between physical and cyber systems. Among those intelligent devices, Android-powered smartphone usage increased significantly due to its low cost and simplicity. But this global prominence of Android operating system also makes it more appealing for cyber-attacks to obtain users' physical private information. Since attackers mostly prefer malicious applications to spread different viruses and take control of the user's device, it is crucial to classify and categorize the malignant application for secure MCPS. Modern machine learning algorithms have shown promising performance in identifying dangerous applications compared to traditional signature-based methods. But most existing works identify only the malicious application where category identification is essential for proper precaution. Also, the static analysis is insufficient for polymorphic malware, which includes regenerating code and changing its properties frequently to evade the detection process. In this study, we compare several state-of-the-art deep learning methods for malapps classification and categorization. Moreover, we propose an ensemble Dynamic Weighted Voting model to identify and label a wide variety of malicious applications using the CCCS-CIC-AndMal-2020 dataset, which contains an extensive collection of Android malware samples. Our proposed ensemble model outperforms the baseline ensemble method Majority Voting by 1% and the classical LSTM model by 2%.
-
Machine Learning Based Soltutions for Automated Priror Learning Assessment and Recognition
Anemily Machina
Artificial IntelligencePrior Learning Assessment and Recognition (PLAR) is a process where people can obtain college credits based solely on their past experiences, outside of formal mechanisms. This process is complicated and time consuming as evaluations are done by hand. Working with the Vector Institute and KnowMeQ during a Vector Fastlane internship my team created a number of machine learning based tools to try and automate the PLAR process and including a working Proof of Concept. The tools automate the majority of the PLAR process, but still leave the final decision to award course credits in the hands of college administrators. This presentation will summarize the results of the project, including the different methodologies and machine learning experiments.
-
Federated Learning with Selective Knowledge Distillation
Gad Mohamed Gad
Distributed Systems and ApplicationsArtificial Intelligence (AI) applications on Internet of Things (IoT) networks often involve relaying generated data to a server for deep learning training, which poses security risks to users' data. Federated Learning (FL) offers a distributed model training paradigm in which local data are kept at the edge and locally trained models are exchanged and aggregated by a server over several rounds to produce a global model. While successful, standard FL algorithms do not support heterogeneous local model design, an essential requirement, especially for resource-limited edge devices. Recently, Knowledge Distillation-based FL algorithms have provided model-agnostic FL to enable clients to independently design their local model and share soft labels instead of model parameters. KD-based FL algorithms are computationally expensive due to additional distillation training. We propose Federated Learning with Selective Knowledge Distillation (FedSKD) to address the limitations of system heterogeneity; and computation and communication demands. We evaluate different aspects of the proposed algorithm relative to baseline FL algorithms. Results show that FedSKD incurs significantly less per-round computation time and communication overhead relative to the considered model-based and KD-based FL algorithms.
-
The Implementation of the Alexander Polynomial of Knots in Maple
Meagan James
Computer AlgebraA knot, in mathematical terms, is an embedding of the circle in three-dimensional space. Knot theory is an area of mathematics which has applications in areas such as biology, robotics, and computer science. One of the primary goals of research in knot theory has to do with determining when two knots are the same. A naive approach to proving that knots are equivalent would simply be to continuously deform one knot until the other is obtained; however, this strategy is not sufficient for proving that two knots are not equivalent. Knot invariants are attributes of a knot that allow us to distinguish between distinct knots. In particular, the Alexander polynomial was the first ever polynomial knot invariant and can be computed using an algorithm that involves labelling a diagram of the knot, constructing a matrix from those labels, and taking a determinant of a particular minor of this matrix. However, it is quite difficult to draw a knot in the math software system Maple. To surmount this obstacle, in this project, I modified an existing knot labelling, called Dowker-Thistlethwaite notation, to uniquely characterize prime knots. From there, I successfully implemented an algorithm in Maple which computes the Alexander polynomial of a knot, given the input of my modified Dowker-Thistlethwaite notation. This means that the Alexander polynomial can be computed almost instantly using this algorithm in Maple.
-
Toward Open-ended Embodied Tasks Solving
Wei Wang
Robotic LearningSequential decision making is fundamental to machine learning and artificial intelligence, which revolves around designing planning and control models that make a series of decisions to achieve a specific goal. An important yet unexplored problem is accomplishing goals that are unforeseen and were not included in the training phase, referred to here as open-ended goals. Our research introduces a novel formulation of open-ended goals as energy functions and presents Diffusion for Open-ended Goals (DOG), a framework capable of zero-shot planning for such goals. In contrast to prior works in which the format and examples of goals were known in training (i.e., the goal in testing is expected), we focus on a setting of training without knowing or using goals and testing with open-ended goals (i.e., the goal in testing can be unexpected). DOG leverages the generative capabilities and the adaptability of training-free guidance of diffusion models to generate sequential plans for any given goal during testing in a zero-shot manner. We evaluated DOG's performance in various tasks, including navigation and robotic movement, demonstrating its effectiveness in achieving novel objectives not covered during its training phase. Our work suggests a fresh perspective on sequential decision-making for open-ended goals.
-
Brain-inspired Adversarial Defence for Computer Vision
Ehsan Ur Rahman Mohammed
Computer Vision and Image AnalysisAdversarial attacks in computer vision are ways in which carefully calculated noise when added to an image results in its misclassification by an AI model. Brain-inspired AI leverages neuroscience research to inform choices while creating AI models. As humans are less vulnerable to adversarial attacks, brain-inspired AI models can be more adversarially robust than traditional AI models. In this presentation, a recent brain-inspired model called V1Net is explored, and it's adversarial robustness is evaluated. Based on other neuroscience insights from existing literature, changes to V1Net that enhances its adversarial robustness are studied. The research is currently in progress hence no research conclusions yet.
-
Efficient Slice Anomaly Detection Network for 3D brain MRI Volume
Zeduo Zhang
Computer Vision and Image AnalysisWhile current anomaly detection methods excel with industrial data, challenges persist in natural images and medical data, where the definition of 'normal' and 'abnormal' can widely vary. Consequently, accurately identifying deviations in structure or function within these types of data remains a critical challenge. Especially for 3D brain MRI data, all the state-of-the-art models are reconstruction-based with 3D convolutional neural networks which are memory-intensive, time-consuming and producing noisy outputs that require further post-processing. We propose a framework called Simple Slice-based Network (SimpleSliceNet), which utilizes a model pre-trained on ImageNet and fine-tuned on a separate MRI dataset as a 2D slice feature extractor to reduce computational cost. We aggregate the extracted features to perform anomaly detection tasks on 3D brain MRI volumes. Our model integrates a conditional normalizing flow to calculate log likelihood of features and employs the Semi-Push-Pull Mechanism to enhance anomaly detection accuracy. The results indicate improved performance, showcasing our model's remarkable adaptability and effectiveness when addressing the challenges exists in brain MRI data. In addition, for the large-scale 3D brain volumes, our model SimpleSliceNet outperforms the state-of-the-art 3D models in terms of accuracy, memory usage and time consumption.
-
Parametric polyhedral set Counting
Yuzhuo Lei
Computer AlgebraThe study of polyhedral sets has numerous applications in mathematics and computer science. One fundamental problem with these sets is the determination of their points with integer coordinates. This question arises in areas like combinatorial optimization (in particular integer linear programming) and optimizing compilers (in particular, the analysis, transformation and scheduling of for-loop nests in computer programs).
In this talk, we will present an algorithm for counting the number of integer points of a parametric polyhedral set. This work is based on the well-known algorithm of Barvinok for integer point counting of non parametric polyhedral sets. We will discuss the challenges in the adaptation of this algorithm to parametric polyhedral sets. We will use our Maple implementation to illustrate our presentation. -
The Delinearization of C programs
Chirantan Mukherjee
Computer AlgebraArray dependence analysis is an essential task in the optimization and parallelization of for-loop nests in computer programs. This analysis relies on a mathematical framework called the polyhedral model. In this model, array references must be affine expressions of the variables in scope (loop counters, array dimension sizes, etc.). However, in some programming languages like C, it is a common practice for programmers to encode multi dimensional arrays by means of one-dimensional arrays. As a result, array references are generally polynomial expressions (with degrees larger than one) of the variables in scope. In order to apply dependence analysis to those latter arrays, optimizing compilers need to transform them into multi-dimensional ones. This latter task is termed "delinearization" in the literature. Today, optimizing compilers perform that task by heuristic methods which may fail in some cases. In this talk, we explain the mathematical problems that must be solved in order to delinearize one-dimensional arrays in C.
-
C3PI: Computational Puzzle for Protein-Protein Interaction Prediction
SeyedMohsen Hosseini
BioinformaticsProteins play crucial roles in cellular functions primarily by interacting with other proteins, making accurate prediction of protein-protein interactions (PPIs) a fundamental challenge. Experimental methods for determining PPIs are often slow and expensive, prompting efforts to enhance computational methods in this domain. In this study, we introduce C3PI, a novel sequence-based deep learning framework designed for predicting PPIs. C3PI adopts an ensemble structure, integrating both 1D and 2D convolutional neural network (CNN) components. Notably, the introduction of a novel "puzzler" component significantly enhances the model's performance. Through comprehensive evaluations across five datasets, C3PI consistently outperforms state-of-the-art programs, particularly excelling in critical metrics such as area under the precision-recall curve (AUPRC) and area under the receiver operating characteristic curve (AUROC). Our findings underscore the substantial improvement achieved by the ensemble model, with the puzzler component contributing significantly to its efficacy.
-
A Comprehensive Framework for Automated Generation of Cloud-Native Applications, Infrastructure, and CI/CD Pipelines
Haoran Wei
Software EngineeringThis research introduces a novel framework aimed at simplifying the development process in cloud computing. Our approach automates the generation of skeleton solutions for cloud-native applications, enabling the provisioning of cloud infrastructures and the creation of continuous integration and continuous deployment (CI/CD) pipeline templates, all encapsulated as code. By generating these foundational elements automatically, our framework provides developers with a head start in their projects, offering them well-structured, customizable templates. This includes basic functional modules of cloud-native applications, optimal infrastructure setups, and predefined CI/CD workflows. The emphasis of this work is on facilitating rapid development cycles and reducing the initial complexity often encountered in setting up cloud-native applications. Our method ensures that the developers have a robust, scalable, and cohesive architectural base, allowing them to concentrate on refining and adding advanced features to their applications. This framework represents a significant advancement in enabling more efficient and streamlined cloud-native application development.
-
Edge Continuum Orchestrator Framework for managing serverless chains across the Cloud-Edge Spectrum.
Muhamed Alarbie
Distributed Systems and ApplicationsThe proliferation of smart devices has paved the way for the development of intelligent yet intricate applications across various sectors. While Cloud Computing offers virtually limitless resources for deploying applications and handling data generated by these devices, emerging applications impose higher performance demands, such as ultra-low latency, high transmission bandwidth, and low energy consumption that surpass the capabilities of traditional IoT and Cloud Computing paradigms. This presentation introduces the Edge Continuum Orchestrator (ECO), a novel framework aimed at addressing the deployment and management challenges of serverless applications across the Cloud Continuum. Through the architectural design and real-world deployment of the ECO, this work presents the performance impacts of cloud-edge continuum infrastructure on serverless chain latency.
-
A Machine Learning Approach to Optimal Lab Test Scheduling Using GEMINI Hospital Data
Joud El-Shawa
Artificial IntelligenceUnnecessary laboratory tests contribute to healthcare waste, leading to increased costs, and risks like hospital-acquired anemia (Cheng et al., 2019). In the context of advancing data-driven healthcare, this research aims to use Long Short-Term Memory (LSTM) networks for real-time prediction of laboratory test requirements, with a focus on efficiently determining the necessity and timing of tests, which are crucial for patient care and resource optimization.
Our research question focuses on applying deep learning techniques to clinical data for predicting whether a lab test occurs. By using LSTM networks, which are useful for modelling sequential data like patient history, we address the gap in real-time healthcare predictions.
We built a machine learning-based system that enhances decision-making for healthcare professionals by predicting the occurrence of glucose tests. We used Python, Keras, Tensorflow, and the GEMINI dataset, which is a large multihospital Canadian healthcare dataset, for development and validation.
Our preliminary results indicate the effectiveness of using LSTMs to predict glucose test occurrences, giving an accuracy of 76%, which is a 23% improvement over our baseline Logistic Regression model. This suggests that including patient history in predictions can improve clinical model performance. Future work involves exploring alternative approaches for data preprocessing and real-world integration.
The novelty of our work lies in the application of LSTMs to model temporal relationships in clinical data for the GEMINI dataset. This research is expected to impact both theoretical and practical aspects of healthcare, streamlining clinical operations and inspiring future artificial intelligence (AI) applications in the field. Additionally, our use of GEMINI data for this application is unprecedented and allows for external validation.
Talks Schedule
Activity | Room | Time |
---|---|---|
Registration | MC312 (Grad Lounge) | 8:00am - 9:00am |
Presentations | MC 105B, MC110, MC316, MC320 | 9:00am - 12:00pm |
Break | - | 12:00pm - 12:15pm |
Keynote | MC110 | 12:15pm - 1:15pm |
Closing Ceremony | MC110 | 1:15pm - 1:30pm |
Lunch | MC312 (Grad Lounge) | 1:30pm - 4:00pm |
Trivia | MC316 | 2:00pm - 2:30pm |
Timetable
Time slot | MC110 Systems and Networks |
MC105B Artificial Intelligence |
MC316 Computer Algebra and Image Analysis |
MC320 Software Engineering and Bioinformatics |
---|---|---|---|---|
9:00AM | Ganyu Wang | Johann Cardenas![]() |
Meagan James![]() |
Chongju Mai![]() |
9:30AM | Gad Mohamed Gad![]() |
Joud El-Shawa![]() |
Christopher Frank Stephan Maligec | Faranak Adinehzadeh |
10:00AM | - | Anemily Machina | Yuzhuo Lei | Haoran Wei |
10:30AM | Muhamed Alarbi | Sabbir Muhammad Saleh | Chirantan Mukherjee | SeyedMohsen Hosseini![]() |
11:00AM | Andrew Bloch-Hansen![]() |
Wei Wang | Ehsan Ur Rahman Mohammed![]() |
Nader Hosseini Naghavi |
11:30AM | Moinul Sayed | Mathias Babin![]() |
Zeduo Zhang | Sabbir Muhammad Saleh |
Judges |
Mostafa Milani Caro Strickland |
Kaizhong Zhang Yalda Mohsenzadeh Mozart Ahadinejad |
Zubair Fadlullah Jim Morey |
Maxwell Yin Elham Bagheri |
Session Chairs | Lucian Ilie | Marc Moreno Maza | Roberto Solis-Oba | Kamran Sedig |
Register now
4th-year undergraduates and graduates can present, anyone can attend. Register Now!
Click to Register