Dan Lizotte
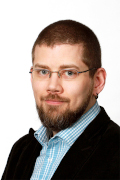
Assistant Professor
Office: Middlesex College 375
Tel:519-661-2111 ext. 86644
Email:dlizotte@uwo.ca
Personal Web Page
Research Group Page: philab.uwo.ca
Social Media: @danlizotte
I am jointly appointed to the Department of Computer Science in the Faculty of Science, and to the Department of Epidemiology and Biostatistics in the Schulich School of Medicine & Dentistry. I am also appointed to the Schulich Interfaculty Program in Public Health and cross-appointed to the Department of Statistics and Actuarial Sciences.
My main research areas are machine learning, biostatistics, and health. In particular, I am interested in machine learning and statistical methodology for supporting health decisions, primarily in public health and primary health care. Many health decisions are made sequentially – for example, health decisions for managing chronic disease are made over time as the disease progresses and as we gain more information about a patient’s health status and about their response to previous decisions. We also gain more information about the evolution of patient preferences as well, which should inform decision-making. Some of my core research work has been in adapting reinforcement learning methodology to be able to support these kinds of decisions that include evolving information and preferences.
Research Interests
Selected Publications
-
Jason E. Black, Jacqueline K. Kueper, Amanda L. Terry, and Daniel J. Lizotte. Development of a prognostic prediction model to estimate the risk of multiple chronic diseases: Constructing a copula-based model using Canadian primary care electronic medical record data. International Journal of Population Data Science, 6(1):1–18, January 2021.
-
Greta Bauer and Daniel J. Lizotte. Artificial intelligence, intersectionality, and the future of public health. American Journal of Public Health, 111(1), January 2021. Opinion Editorial (Peer reviewed).
-
Pananos, A. Demetri and Daniel J. Lizotte. Comparisons between Hamiltonian Monte Carlo and maximum a posteriori for a Bayesian model for apixaban induction dose & dose personalization. In Finale Doshi-Velez, Jim Fackler, Ken Jung, David Kale, Rajesh Ranganath, Byron Wallace, and Jenna Wiens, editors, Proceedings of the 5th Machine Learning for Healthcare Conference, volume 126 of Proceedings of Machine Learning Research, pages 397–417, Virtual, 07–08 Aug 2020. PMLR.
-
Jason E. Black, Amanda L. Terry, and Daniel J. Lizotte. Development and evaluation of an osteoarthritis risk model for integration into primary care health information technology. International Journal of Medical Informatics, 141:104160, 2020.
-
Jacqueline Kueper, Amanda Terry, Merrick Zwarenstein, and Daniel J. Lizotte. Artificial Intelligence and primary care research: A scoping review. The Annals of Family Medicine, 18:250–258, May 2020.
-
Daniel J. Lizotte, Mayuri Mahendran, Siobhan M. Churchill, and Greta R. Bauer. Math versus meaning in MAIHDA: A commentary on multilevel statistical models for quantitative intersectionality. Social Science & Medicine, 245:112500, 2020.
-
Davis, Brent D., Kamran Sedig, and Daniel J. Lizotte. Archetype-based modeling and search of social media. Big Data and Cognitive Computing, 3(3), 2019.
-
Maria Jahja and Daniel J. Lizotte. Visualizing clinical significance with prediction and tolerance regions. In Finale Doshi-Velez, Jim Fackler, David Kale, Rajesh Ranganath, Byron Wallace, and Jenna Wiens, editors, Proceedings of the 2nd Machine Learning for Healthcare Conference, volume 68 of Proceedings of Machine Learning Research, pages 217–230, Boston, Massachusetts, 18–19 Aug 2017. PMLR.
-
Daniel J. Lizotte and Arezoo Tahmasebi. Prediction and tolerance intervals for Dynamic Treatment Regimes. Statistical Methods in Medical Research, 26(4):1611–1629, 2017.
-
Daniel J. Lizotte and Eric B. Laber. Multi-objective Markov decision processes for data-driven decision support. Journal of Machine Learning Research, 17(211):1–28, 2016.
-
E. B. Laber, D. J. Lizotte, M. Qian, W. E. Pelham, and S. A. Murphy. Dynamic treatment regimes: technical challenges and applications. Electronic Journal of Statistics, 8(1):1225–1272, 2014.
-
Daniel J. Lizotte, Michael Bowling, and Susan A. Murphy. Linear fitted-Q iteration with multiple reward functions. Journal of Machine Learning Research, 13:3253–3295, Nov 2012.
-
Daniel J. Lizotte. Convergent fitted value iteration with linear function approximation. In J. Shawe-Taylor, R.S. Zemel, P. Bartlett, F.C.N. Pereira, and K.Q. Weinberger, editors, Advances in Neural Information Processing Systems 24, pages 2537– 2545. NIPS Foundation, 2011.
Teaching
-
COMPSCI 4414/9114/9637 – Introduction to Data Science
-
MPH 9017 – Public Health Informatics
-
COMPSCI 4417/9117/9647
Professional Activities and Industry Links
-
Alliance for Healthier Communities https://www.allianceon.org
-
PointClickCare https://pointclickcare.com